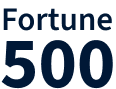
A leading semiconductor producer enhanced their yield using our technology. This producer’s struggle with yield losses mirrors a broader challenge in the industry.
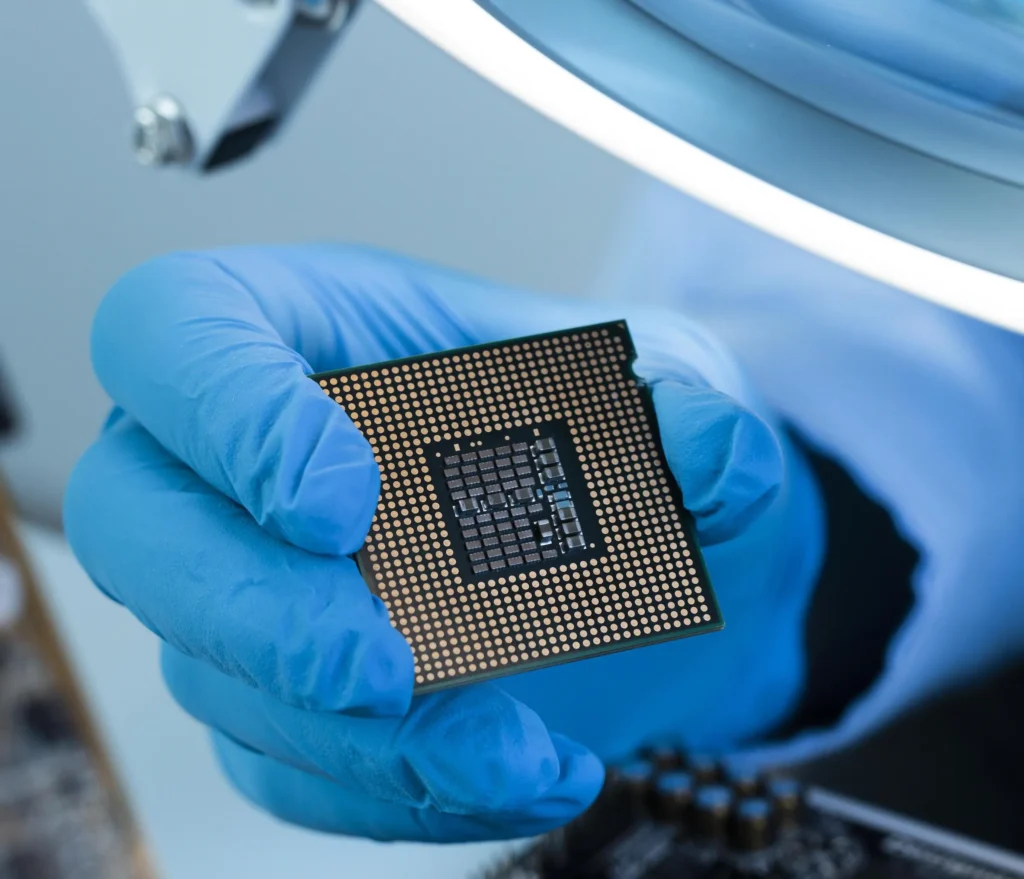
Industry
Electronics
Location
Europe
Product
Analyst
Over the past years, several semiconductor producers have lost customers due to yield problems. While producers tend to be hesitant to disclose specific yield figures, industry estimates commonly place these yields between 85% and 95%. Yield losses are particularly pertinent for new product generations, where recipes and production parameters must be calibrated from scratch. Consequently, determining the root causes of yield losses is not only a primary concern but also a potential competitive edge in the semiconductor industry.
Semiconductor manufacturing has long leaned on statistical methods for quality improvement. Many existing approaches are based on linear methods, such as the correlation analysis between equipment routing features and yield. However, these linear methods often fail to detect nonlinear relationships or interaction effects between process parameters. Overlooking these complexities, especially in manufacturing settings with large data quantities, can easily lead to missing the root causes of yield losses.
To address the shortcomings of linear methods, many producers have recently turned to data analysis based on conventional machine learning (decision trees, random forests, etc.). But there is an inherent limitation with these methods: they are designed for predicting quality outcomes rather than improving them. When assessing feature importance, machine learning algorithms can overfit the underlying data, which leads to misleading conclusions. They might assign high importance to variables that are simply correlated to yield issues, as opposed to being causally related. At the same time, critical process parameters influencing yield might be neglected, as these methods do not account for the sequential and hierarchical nature of the manufacturing processes.
Facing these challenges, our case company encountered these very limitations, struggling to pinpoint the root causes of its quality losses using both linear methods and conventional machine learning algorithms.
Senior Product Engineer
The initial deployment focused on a new generation of semiconductors. The company selected this product due to its comparably low yield for which the root causes remained unclear. The process engineers at the company merged three data sets for their analysis: (1) equipment routing data (timestamps, equipment IDs, chamber IDs, etc.), (2) within-process measurements (layer thickness, equipment pressure, etc.), and (3) batch-level yield data. Using our AI tools, the process engineers uncovered previously unrecognized relationships in an etching process that were linked to significant yield losses. Based on these newfound insights, the process engineers implemented corrective measures such as recalibrating the etching equipment, and ran experiments to validate their effectiveness. The efficacy of the proposed improvement measures was experimentally verified and then monitored over a longer time period.
Senior Product Engineer
Overall, the producer witnessed a reduction in yield losses by 51.3%. These remarkable improvements are not only a testament to the capability of our technology, they also highlighted the shortcomings of traditional tools for quality analysis. Notably, the suspicious processes identified by our tools were overlooked by conventional industry methods. Today, the company has redesigned their analytics pipeline and redefined their approach to quality improvement.
In an industry where semiconductor manufacturers often face yield losses of 10% to 15%, it is crucial to make the most of their data. Achieving a high yield and ensuring a stable supply not only offers a competitive advantage, it also builds trust with customers. Implementing the right AI tools brings about a significant economic impact, ensuring that manufacturers can maintain the highest standards of quality while optimizing their operational efficiencies.
Impact
Causal AI beats established methods
No Blind Spots
Process experts had overlooked an implausible equipment issue in an etching step. Our technology quickly pinpointed it.
Actionable Insights
The findings directly lead to improvement actions that worked. Yield losses were reduced by 51.3%.
Structural Improvements
Thanks to this experience, this fab also redesigned its analytics pipeline and changed its approach to quality improvement.