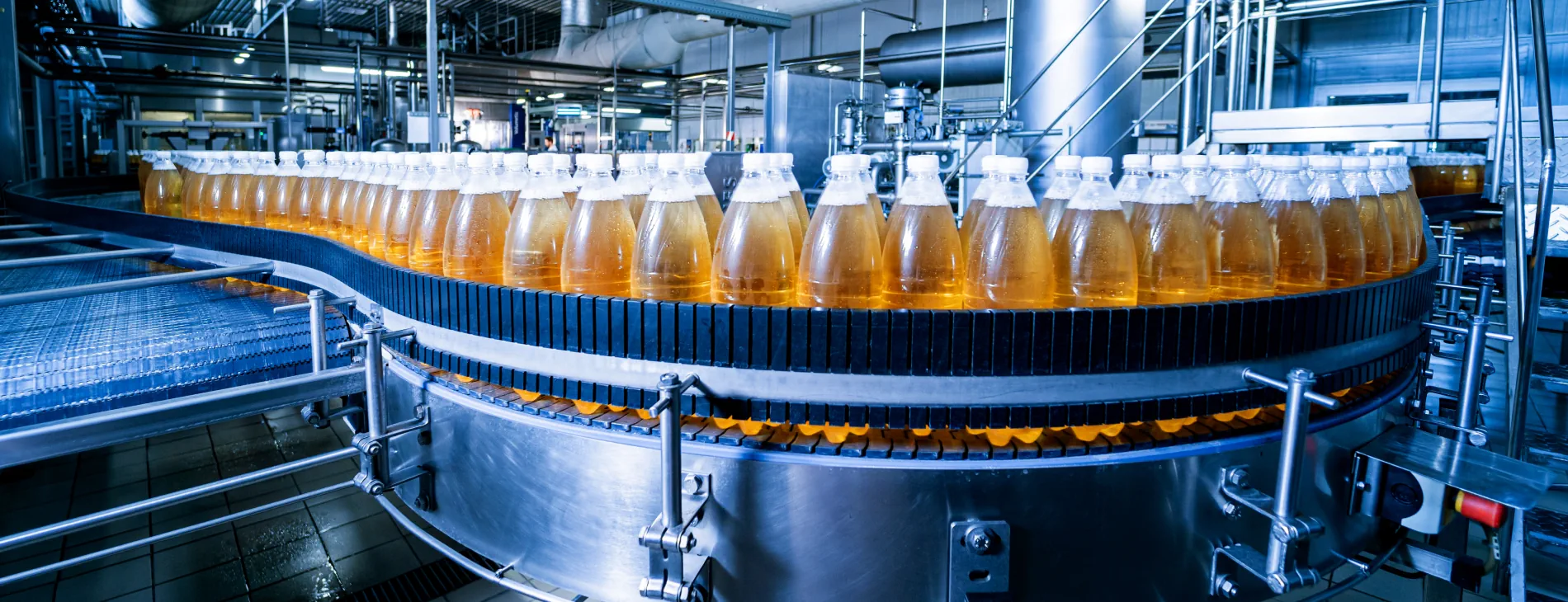
Blog
AI is changing how expert knowledge is used in manufacturing
In manufacturing, troubleshooting production issues through changes to the product, processes, or equipment requires skilled engineers who know exactly what they are doing. Artificial intelligence (AI) is unlikely to fully replace them any time soon. However, the way in which these experts work is changing considerably already today.
Eliminating human bias
Today, the process of identifying the root cause of a production issue often starts with a brainstorming session among experts. Engineers would sit together and discuss the most likely failure mechanisms. Next, they would collect data to prove or reject their hypotheses. AI-based analytics flips this process around. Data are continuously collected, the AI identifies the most likely root causes, and THEN the engineers sit together, interpret the results and decide how to move on with trials or improvement actions.
This new workflow has one major advantage: it removes the engineer’s bias to a large extent. While humans would intentionally or non-intentionally confine their search for the root cause to a limited scope of relations that they consider probable, algorithms will search the entire parameter space covered by the available data. The more tricky issues with unexpected root causes will be found by the AI, but might slip through the cracks in a traditional troubleshooting effort. This considerably shortens the time between detection of a problem and its resolution.
In a recent collaboration with a semiconductor manufacturer, we were faced with a yield drop that caused considerable financial loss. Even after two weeks of production under these unfavorable conditions, the process experts were still in the dark about where the problem could come from. Their traditional methods for root cause analysis didn’t seem capable of tracking down the problem. Although they had semi-automated routines to browse through a large amount of data, they couldn’t find any convincing relationships between process data and quality.
When we stepped in with our AI-based approach, we soon found a relationship that had very high statistical significance. However, the relationship seemed physically implausible. It concerned a process step that was outside of the expert’s focus because at that stage of manufacturing, the product was believed to be sufficiently protected against detrimental effects. Nevertheless, our feedback was taken into consideration, and some trials were done that confirmed that the problem arose exactly in the process we pointed at. The engineers were able to fix the issue although the physical failure mechanism was only understood much later. Using our algorithm, we were able to considerably shorten the time between detection and resolution of the issue. This shortened the period of low-quality production and saved more money than needed to justify the investment in AI with only a single incident.
This example shows how AI can overcome a crucial limitation of traditional troubleshooting in manufacturing: humans are only looking where they expect to find something. Whenever something unexpected happens, this bias can lead to failure and long delays in the problem-solving process.
Workflow evolution: The role of expert knowledge in the AI era
The example also shows that there is a shift in how expert knowledge enters the game: Instead of spending most of their time with identifying the most probable root causes, extracting data from various systems, joining tables, and finding complex relationships, the daily tasks of experts shifts towards evaluating results from the AI-based analytics tools and translating them into practicable improvement actions.
That is how it works in an ideal world …
In reality, things are a bit more complicated. In order to unfold their full potential in finding root causes, AI-based analytics tools need high-quality data. Collecting such data requires expertise, as well. Every sensor that collects useful data for root cause finding must get installed by a human. Which kind of sensor do we put where? This kind of decision-making cannot be done by a machine in the foreseeable future. Most sensors that we currently find in process equipment were primarily installed to monitor the health of the equipment or the conditions of the process. This kind of data often relates to product quality in some way or another and can be useful in finding root causes. However, the more we target the measurements directly at product quality the more powerful the data become for AI-assisted tools. A process expert who has knowledge of all past incidents and improvement actions can incorporate this knowledge into the data collection and, thereby, make sure that similar issues will pop up early and get fixed before they even result in relevant scrap rates.
Conclusion
In summary, we observe that AI is changing the way in which experts in manufacturing use their knowledge. Instead of doing a “manual” analysis on data they collected based on what they know to be a probable root cause, they let AI-based analytics tools do the analysis for them and use their knowledge to (1) feed these tools with the most valuable data they can get and (2) translate the AI results into useful improvement actions.